It’s no secret that generative AI has brought many benefits to industries and teams worldwide. From generating potential drug compounds in healthcare to supporting the creation of high-quality design assets for marketing departments, it seemingly uprooted businesses from their status quo and created a new normal in the blink of an eye.
But while its uptake was swift and enthusiastic for some, not everyone welcomed generative AI with open arms. Some worried that it was too good to be true, prompting them to dig deeper into the ethics of generative AI. As a result, generative AI ethics has become a hot topic of conversation, with AI moral dilemmas getting increasingly scrutinized.
To help you get a better understanding of the generative AI dilemmas and form a more well-rounded opinion on the good and bad of generative AI, we’ll be answering one of the most pressing questions in the age of artificial intelligence: “What are some ethical considerations when using generative AI?”
In doing so, we’ll break down the nine key ethical problems of generative AI you should be aware of.
Table of contents
- AI biases and stereotyping
- Content ownership and copyright issues
- Environmental impact of AI
- Deepfakes and synthetic media
- AI hallucinations and false information
- Transparency in AI decision-making
- Accountability of AI models
- Loss of humanity in art
- Job displacement and workforce impact
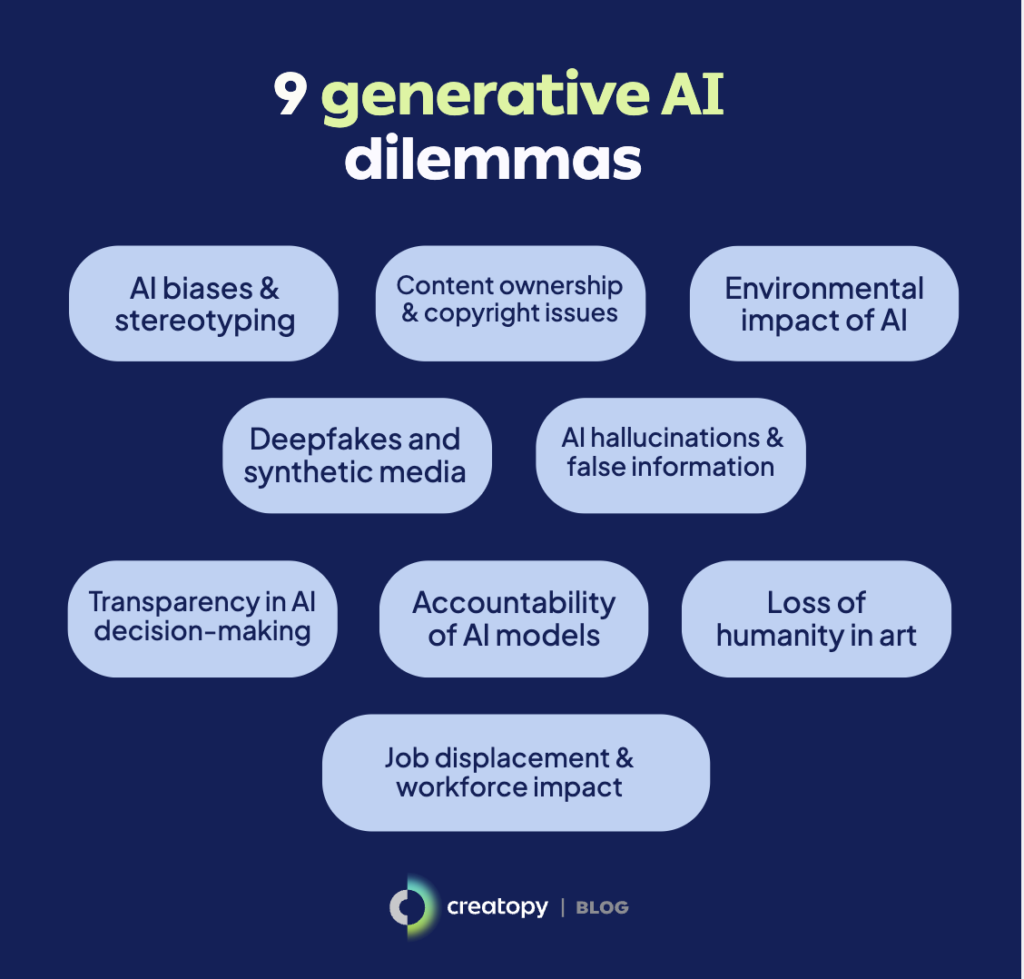
1. AI biases and stereotyping
Humans, by nature, tend to rely on patterns found in past experiences and use these to form predictions. AI models function similarly: They’re trained on a large dataset, evolve by identifying statistical patterns, and generate outputs based on learned correlations.
A major difference is that generative AI models are built on massive datasets of human content. On top of this, they can’t actually “think” for themselves. They don’t possess independent reasoning or a genuine understanding of the subject at hand, they merely recognize and recreate human thought patterns.
Unfortunately, these human thoughts aren’t always impartial. They often present skewed ways of thinking shaped by stereotypes, racial and gender biases, and ideological leanings. This is one of the many AI and ethical issues that make AI models controversial—and an increasing number of studies are starting to corroborate this.
One study assessing gender bias in commercial image recognition systems found that images of women received three times more annotations focused on their physical appearance than images of men. In contrast, the labels applied to men emphasized class and professional status.
While it’s not necessarily wrong for AI to detect a smile in women or label a man as a businessperson, the reverse is just as true—a woman can be recognized as a businessperson, and the man is smiling, too. However, AI detection mechanisms fail to capture this balance, potentially reflecting the biases embedded in their training data.
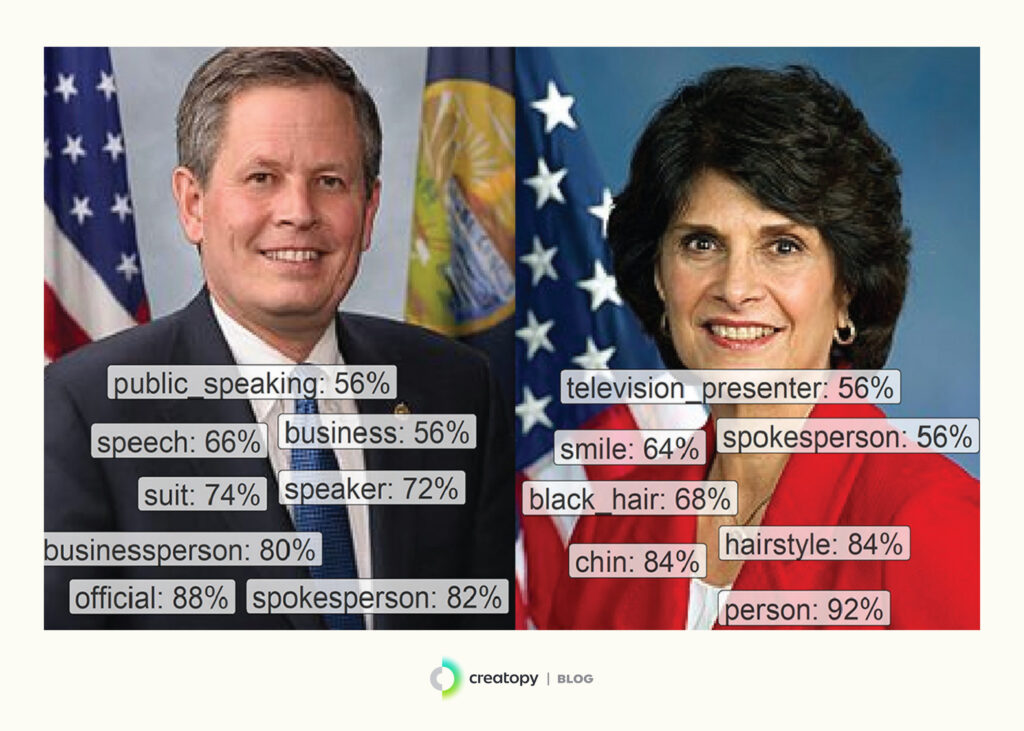
Another study by USC researchers revealed that up to 38.6% of AI systems’ “common sense” statements are biased, with women being described more negatively than men, Muslims being associated with terrorism, Mexicans being associated with poverty, and so on. These biases aren’t just offensive—they can actually affect human lives: AI decision-making systems are gradually seeping into areas such as criminal sentences, already influencing outcomes in states like Arizona and Colorado.
AI’s entire reason for existing is that they identify patterns and use them to make predictions. Sometimes it’s very helpful—like they see a change in atmospheric pressure and predict a tornado—but sometimes those predictions are prejudiced: they decide who to hire for the next job and overgeneralize from prejudice that is already in society.
—Jay Pujara, USC Viterbi research assistant professor of computer science
2. Content ownership and copyright issues
The second issue is ownership—the gray area surrounding who owns the content produced by AI models. As mentioned, generative AI models process and replicate patterns found in existing content, some of which are proprietary. This raises the legal and ethical dilemma in AI of content being used without permission from the original creators.
In traditional settings, this is clearcut. If filmmakers want to use an artist’s song in a movie, they must obtain a licensing agreement from the rights holder. Without this agreement, using their song in the film would clearly and irrevocably be considered theft or infringement under intellectual property law.
However, things aren’t so black and white when it comes to AI.
Since AI models are often trained on vast amounts of copyrighted materials, attribution is difficult. It’s unclear which artist’s work contributed to any given output. On top of this, AI companies argue that using proprietary data is okay, given it’s being used for pattern recognition and not replication, equating it to how humans learn by reading books.
They therefore defend their stance under “fair use,” which permits using copyrighted material for education, commentary, or research purposes. This has led to one of the biggest artificial intelligence ethical dilemmas faced by courts today, with judges at a standstill as they grapple with whether AI training on proprietary data qualifies as fair use or constitutes outright infringement.
3. Environmental impact of AI
As the use of generative AI tools grows, so do sustainability concerns—particularly regarding its substantial energy use, CO2 emissions, and water consumption. Every stage of an AI model’s development, from training to deployment, leaves a significant environmental footprint.
Just take a look at these statistics:
- Training a medium-sized generative AI model can demand 626’000 tons of CO2 emissions, equal to the lifetime emissions of five average American cars.
- It’s estimated that a person who engages in a session of questions and answers with GPT-3, which typically spans 10-50 responses, consumes half a liter of fresh water to keep data centers cool.
- A ChatGPT query requires nearly 10 times more electricity than the average Google search.
Governments are racing to develop national AI strategies but rarely do they take the environment and sustainability into account. The lack of environmental guardrails is no less dangerous than the lack of other AI-related safeguards.”
– Golestan Radwan, Chief Digital Officer of the United Nations Environment Programme (UNEP)
4. Deepfakes and synthetic media
Deepfakes combine machine-learning algorithms with facial-mapping software to insert data into digital content—sometimes without explicit permission.
The technology dates back to the 1990s when researchers used computer-generated imagery to create realistic human images. It has since evolved, with massive breakthroughs in machine learning and large datasets powering more sophisticated deepfakes in the 2010s. The term itself, however, was coined in 2017 by a Reddit user who created a subreddit for illicit face-swapping materials focused on celebrity videos.
While distinguishing the real from the fake used to be a no-brainer given the lower quality of older deepfakes, that is hardly the case today. AI technologies have made deepfakes more convincing than ever, making it nearly impossible to tell a deepfake from the real thing.
And while some use deepfakes for fun, like seeing their aged selves or animating old photos, others use them maliciously. Texts, videos, and audio clips can be altered to make people look like they said or did something they, in fact, hadn’t. If these deepfakes are believable enough, they can have grave consequences.
In 2024, a British multinational company reported a $25 million loss after fraudsters used deepfake technology to impersonate the company’s CFO and convince employees to authorize large fund transfers. And this is not an isolated case. Plenty of other deepfake incidents are reported almost daily, as seen in Resemble.AI’s deepfake incident database below.
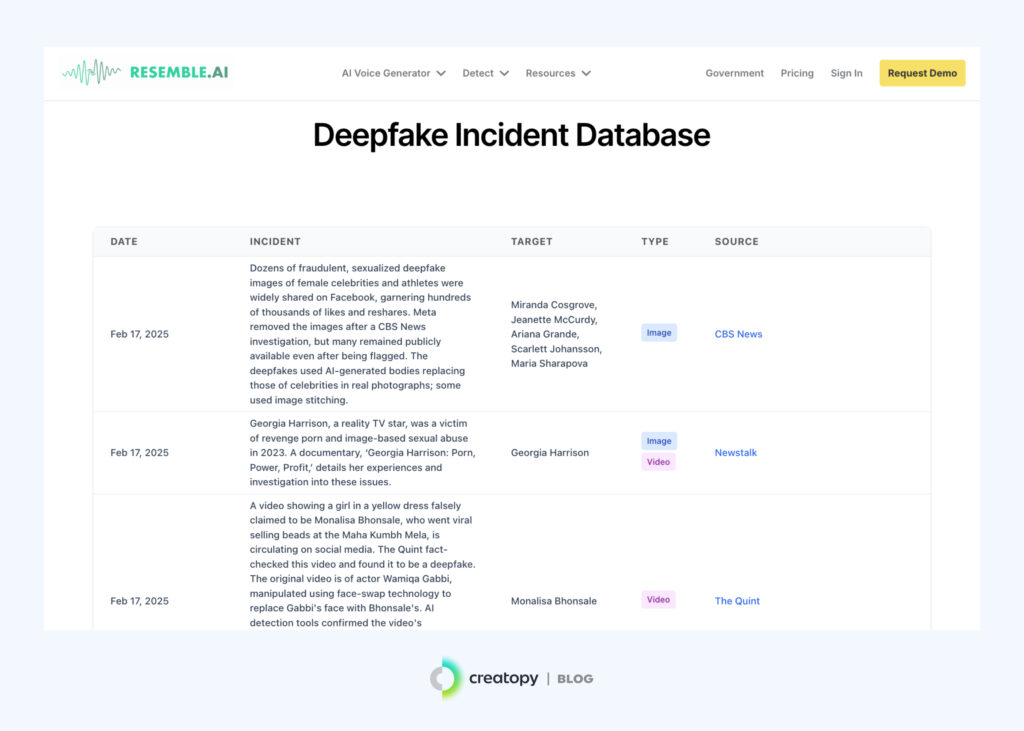
5. AI hallucinations and false information
Generative AI “hallucinations” and AI-generated answers go hand in hand. Despite their advanced capabilities, AI models often produce plausible-sounding—albeit incorrect—outputs and present these as facts. Since the incorrect information can sound very realistic, there’s an inherent risk of widespread deception, as with deepfakes.
For example, Meta AI introduced Galactica in November 2022, an AI model built to support scientific knowledge. Yet, the model often cited non-existent research papers, causing its withdrawal just two days after its release. More recently, Google Gemini AI’s Super Bowl ad in February 2025 showcased an erroneous statistic about cheese generated by AI, which claimed that Gouda accounts “for 50 to 60 percent of the world’s cheese consumption.”
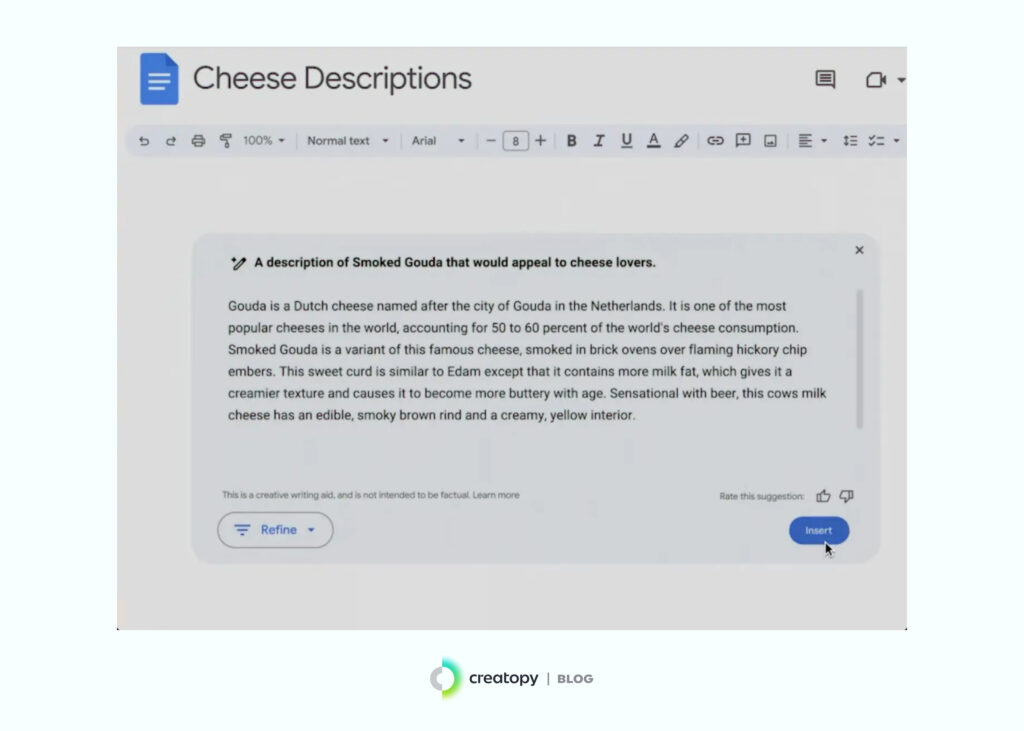
These errors stem from the foundational technologies on top of which AI models are built. At their core, they’re probabilistic algorithms that, rather than possessing proper understanding or reasoning capabilities, merely predict the most statistically likely sequence of words for any given input.
6. Transparency in AI decision-making
Another challenge of generative AI is the lack of transparency into how it produces its outputs. This issue has come to be known as the “black box problem,” which creates an AI dilemma for businesses and regulators alike.
Firstly, we don’t know how AI models make decisions. While this may seem harmless when generating an answer to a question such as “What’s a fun fact about penguins?” It’s not so innocuous in the case of autonomous AI-powered vehicles.
If, say, an AI-powered vehicle hits a pedestrian instead of allowing them to cross, our lack of visibility into the AI model makes understanding the system’s thought process and decision path difficult. As a result, diagnosing errors and refining AI decisions for safer performance becomes a challenge.
Secondly, we can’t assess or mitigate biases if we don’t know what data was used to train AI models. If AI models are trained with data rife with biases, flawed assumptions, or representation gaps, the generative AI model will inevitably regurgitate these in its outputs.
Lastly, there’s the issue of trust: How can we trust something we don’t have complete information on? Without clear explanations of how AI produces its outputs—a practice known as “explainable AI”—AI adoption and growth can remain stunted, held back by skepticism and regulatory pushback.
7. Accountability of AI models
Transparency into generative AI models is just one aspect of understanding how they work. Determining who should be held responsible for their impact is just as important. This dilemma is best illustrated by the AI trolley problem—a modern take on the classic ethical thought experiment.
If AI were driving an autonomous vehicle and it had to decide between swerving to avoid a pedestrian and potentially harming the passenger, or continuing forward to injure the pedestrian instead, who would be accountable for that decision? Is it the car manufacturer tasked with implementing the AI model? The software developers who programmed the AI model’s risk assessment? Or the vehicle owner who entrusted AI technology with driving?
The vast ecosystem of AI creators, users, and regulators makes it challenging to default responsibility to a particular person or institution. Here’s why:
- Developers and AI model creators: AI models result from collaborative efforts over time, making assigning individual blame a problem. Couple this with developers’ lack of direct control over how AI models respond in the real world post-deployment, and you’ll realize developers make unsuitable scapegoats.
- End users: Holding end users responsible for AI’s outputs assumes they have complete control over AI models’ outputs and fully understand how they work. This is certainly not the case due to AI’s black box nature, which makes it difficult to interpret how and why an AI model reached a particular decision.
- Data providers: While the quality of AI training data can influence AI models’ decisions, holding data providers responsible isn’t an option. Data often reflects natural human tendencies and biases, meaning biases should be seen as an unavoidable reality when it comes to human data, not malpractice. Additionally, the sheer amount and complexity of data makes handpicking bias-free data impractical and unrealistic.
- Regulatory bodies: Some blame policymakers for weak AI regulations, but excessive restrictions could stifle innovation. As AI continues to evolve, regulators are still trying to find a balance—the sweet spot—between oversight and progress, ensuring one doesn’t outweigh the other as AI models continue to transform.
8. Loss of humanity in art
Art requires original thinking based on lived human experiences. It’s a byproduct of expression—a channel for humans to understand, explore, and communicate their life stories. Generative AI tasks now assume this role but cannot actually embody it.
This raises a key AI ethical dilemma: Can something really be considered art if it’s generated by AI, without human emotion or lived experiences fueling its creation?
While AI is great at taking on repetitive, predictable tasks, its transition into creative spaces raises concerns, specifically about AI stripping art of its humanity, depth, and meaning. Simply put, generative AI lacks the human qualities to turn “output” into “art.” It merely mimics artistic styles and reworks learned inputs to match user prompts.
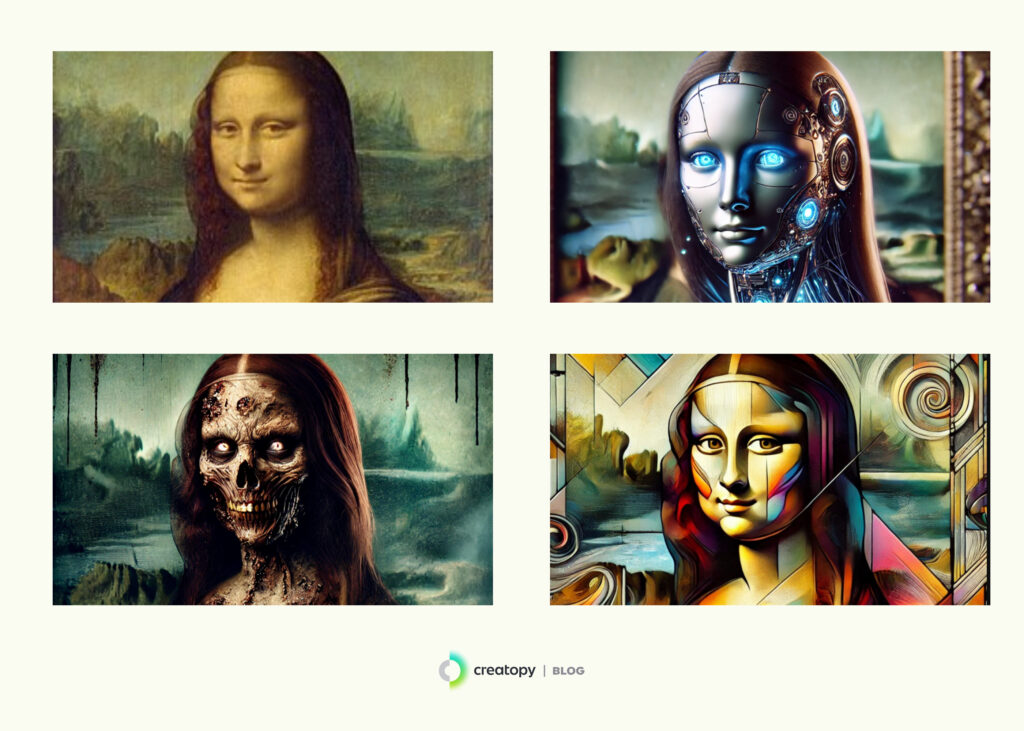
As Molly Crabapple, award-winning artist, writer, and author, crudely states, “Generative AI is vampirical—feasting on past generations of artwork even as it sucks the lifeblood from living artists. Over time, this will impoverish our visual culture.”
9. Job displacement and workforce impact
The last generative AI ethics issue we’ll discuss is its impact on the job market. While some may argue that AI has created new jobs, such as prompt engineering, most worry that it will create an avalanche of job displacements. After all, a 2023 Goldman Sachs analysis revealed that generative AI has the potential to automate up to 300 million full-time jobs in the US and Europe.
Therefore, most decision-makers are left treading an uncomfortable middle ground. They acknowledge that AI allows employees to offload mundane and mind-numbing tasks. At the same time, they may be tempted to automate entire job roles to reduce costs, using it to replace rather than assist workers.
Workers have already taken retaliatory action in fear of this potential reality. For example, the Writers Guild of America (WGA) strike in 2023 highlighted concerns that AI-generated content could replace human scriptwriters in the entertainment sector.
The impact of gen AI alone could automate almost 10 percent of tasks in the US economy. That affects all spectrums of jobs. It is much more concentrated on lower-wage jobs, which are those earning less than $38,000. In fact, if you’re in one of those jobs, you are 14 times more likely to lose your job or need to transition to another occupation than those with wages in the higher range, above $58,000, for example.
—Kweilin Ellingrud, McKinsey Global Institute Director and Senior Partner
Is generative AI the right choice for you?
Generative AI is a paradox for regulators, companies, and users alike. On the one hand, it undoubtedly makes certain parts of our lives and jobs easier. At the same time, it adds an unprecedented layer of complexity, disrupting the norm with risks to job security, artistic expression, and the truth itself while opening up gray areas of accountability, transparency, and ethical problems with AI.
As with most things, we must avoid swaying too far to one side. Letting AI take the reins entirely without much thought dilutes the human element needed to connect with target audiences. On the other hand, rejecting generative AI can reduce a company’s effectiveness, leaving it trailing behind competitors that have thoughtfully adopted it as part of their workflows.
At Creatopy, we believe AI should support, not replace, human creativity—and our creative automation platform is built with this philosophy at its core. Rather than letting AI take the lead, you can use Creatopy’s AI to support designers and non-designers across the design workflow while maintaining creative control. How? By leaning on AI during the key stages where efficiency is paramount while keeping human input central to all actions. This human-led, AI-supported approach drives innovation without risking the loss of the authenticity only people can bring.
The best results come from partially integrating generative AI into some—not all—business processes, using it as a supplementary driving force rather than something that completely overhauls your operations. If you’re unsure where to start, here are 11 use cases for AI in design workflows you can use as a segue into generative AI adoption.